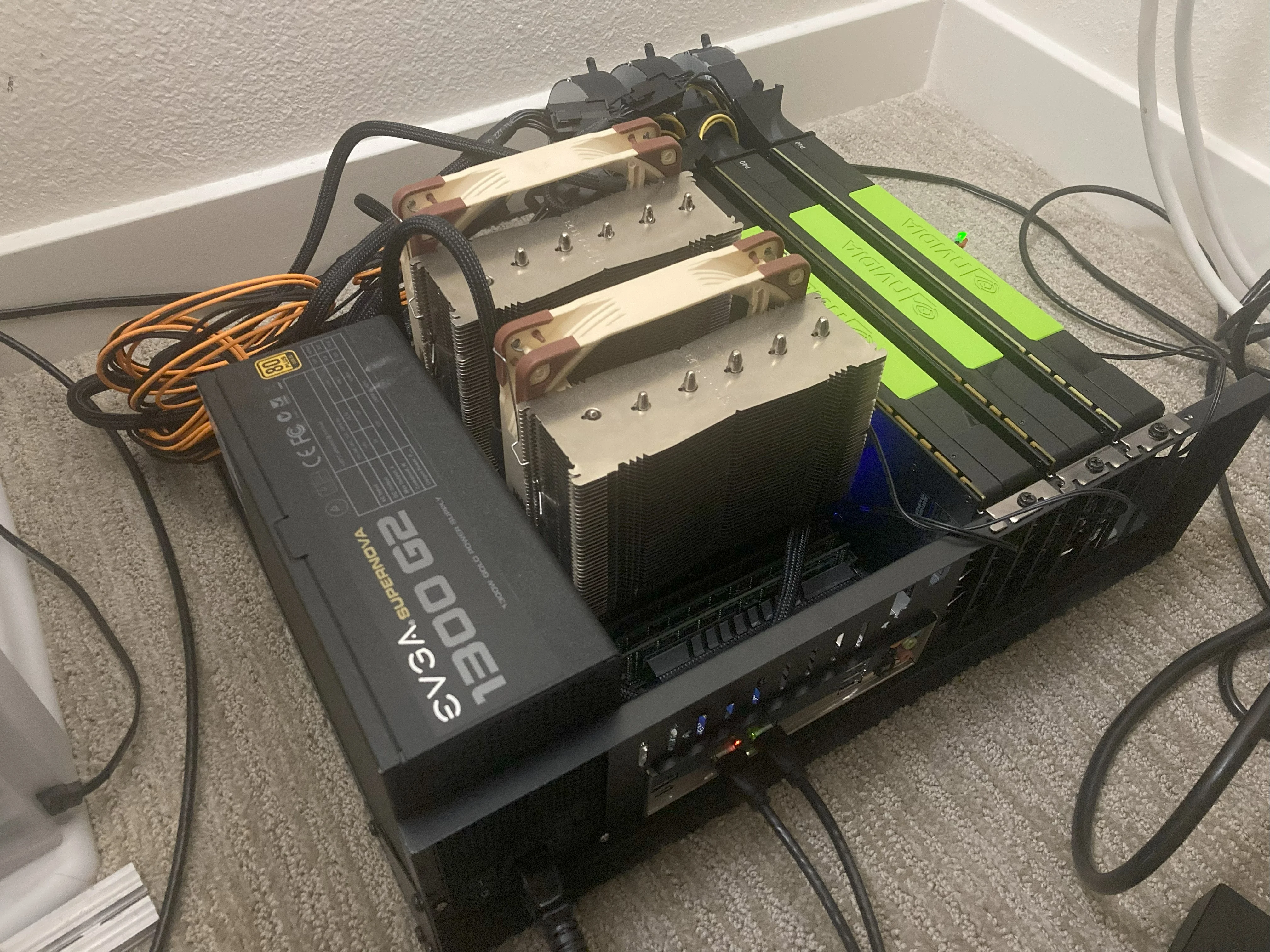
The cheapest deep learning workstation
Introduction
Let’s suppose that you are a deep learning enthusiast.
You want to test a new fancy open source model like speech2text, text2speech, txt2img or event txt2video.
What should you do ?
- 💁 - Pay for different platform for each of your tests
- ☁️ - Rent a cloud instance by the hour
- 💻 - Use a Google Colab notebook and hope to get a GPU
- 📟 - Run it on your own hardware
I’m personally in favor of the latter, as you learn more. But the main challenge is the cost of the hardware.
In this blog post, I will reveal my secret to build the cheapest deep learning workstation you have ever seen.
The formula
The formula is simple : buy used parts on Ebay or LeBonCoin.
I can offer several declinations
Cheapest formula
40€ motherboard + CPU + RAM combo
9€ power supply
96€ K80 GPU
Budget deep learning workstation
145€ for our budget setup
vs
168€ for 7 days of full time g5.xl time
Declination 2 - The SCALING ™️ formula
Goal : upgrade the GPU to a P40 or multiple ones.
170€ can be the cost of your P40 GPU.
Because it is seen as a single 24Gb gpu and not multiple ones like the K80, the P40 is a better choice for loading LLMs.
With multiple GPUs, you can approach the price of more expansive instances like the [g5.12xlarge]
Scaling deep learning workstation
110€ motherboard + CPU + 128gb RAM combo
46€ 1300w power supply
410€ : 3 x P40 GPU
Price : 560€
Price comparison - an AWS reference
We will use the prices from AWS to compare how cheap our deep learning workstation is.
7 days of g5.12xlarge = 953€.
We assume we pay for only 3 GPUs = 715€
So, our SCALING workstation is already 22% cheaper than renting on AWS for a 3 GPU instance.
What it looks like
The result can also be eye pleasing. You can also buy a case, if you don’t like the mess.
Not mine, but a good point of reference.
The catch
These numbers are unfortunately hiding a few things :
- 🔋- Power consumption : it will be hot in the room hosting your new machine
- 📉 -Lower performance : the GPU are a bit outdated, inference performance will be below the professional cards
- 🏃- Training : you may consider another setup if you want to train a large model, as it will be too slow and energy hungry
But….
We compared to a g5 instance, which packs a A10 GPU, very far from state of the art
- The A100 is a reference for enterprise grade deep learning (but is extremely costly)
- On some benchmarks, the A10 is 2x slower than the A100 (on stable diffusion)
- But the A10G is still 50% above the P40
- But when comparing with a 500€ consumer GPU card, the A10G would be 30% below the 4060 ti which is a 500€ card
All in all, renting a cloud GPU is not a very good deal if you are not a company.
Appendix
The sources I used to build this article :
- The local llama sub-reddit : https://www.reddit.com/r/LocalLLaMA/
- A comparison of the different GPU options